Massachusetts prevalence of opioid use disorder estimation revisited: Comparing a bayesian approach to standard capture-recapture methods
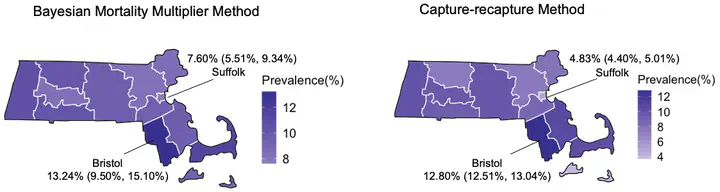
Abstract
Accurate estimates of opioid use disorder (OUD) are important for resource allocation and system planning. Indirect estimation methods may provide more accurate estimates than surveys. Using 2015 data from the Massachusetts Public Health Data Warehouse (PHD), we performed a comparative analysis of two indirect estimation methods to estimate OUD prevalence among the Massachusetts Medicaid population. We first used a seven data set capture/re-capture (CRC), stratified by age, sex, and county to account for the individual heterogeneity. We used log-linear models to estimate the unknown OUD population and estimated confidence intervals (CI) using distributional bootstrapping. We applied a Bayesian prevalence estimation model by linking Medicaid claims data to opioid-related death data with the assistance of OUD treatment data. In doing so, we were able to calculate opioid-related death rates for the known OUD population while off-treatment. We assumed that unknown persons with OUD had overdose death rates equal to the known population while off-treatment, following a Poisson distribution. We added random effects to counties and generated 95% credible intervals (CrI) from posteriors. The prevalence of OUD was 9.43% (95% CrI: 7.49%, 12.00%) in the Bayesian model and 8.60% (95% CI: 8.57%, 8.65%) in the CRC. Estimates in small counties were more different than large counties. Estimates were more similar among middle-aged groups than older or younger age groups. From this analysis, we found that the CRC, with more data available, provided a similar state-level estimates to the Bayesian method; but the group-specific estimates of unobserved OUD differed.